Mean models —
Analyzing historical simulations shows hottest models aren’t the most accurate.
Scott K. Johnson
–
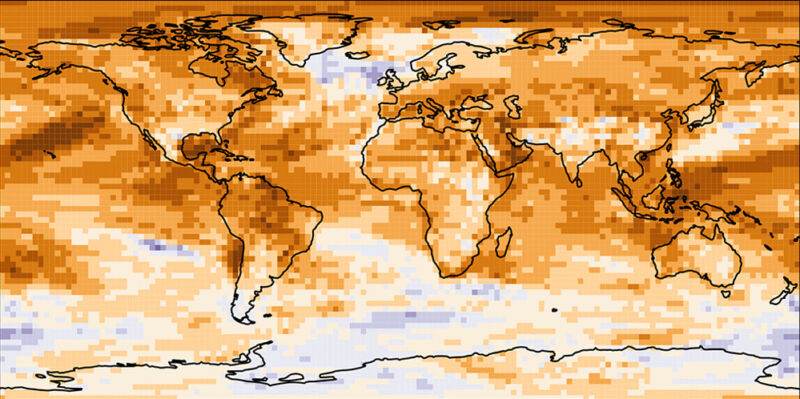
One notable storyline in the climate system over the past year or two has been the effort to make sense of the latest generation of climate models. In service of the next Intergovernmental Panel on Climate Change (IPCC) report, the world’s climate models have submitted their simulations to the latest database, known as CMIP6. These submissions showed that updates to a number of models had made them more sensitive to greenhouse gases, which means they project greater amounts of future warming.
Apart from diagnosing the behavior responsible for that change, climate scientists have also wrestled with the implications. Should we be alarmed by the results, or are they outliers? Climate models are only one tool among many for estimating Earth’s true “climate sensitivity,” so their behavior has to be considered in the full context of all the other evidence.
For a number of reasons, research is converging on the idea that the high temperature projections are outliers; these hotter models seem to be too hot. That will present a challenge for the scientists working on the next IPCC report: how much influence should these outliers have on projections of future warming?
Weighting game
One way to represent the range of uncertainty in projections is to simply average all the available model simulations, bounded by error bars showing the highest and lowest simulation. This is an agnostic solution that doesn’t attempt to judge the quality of each model. But another approach is to use a weighted average, scoring each model in some way to generate what is hopefully a more realistic projection. That way, adding several versions of a model that gets drastically different results, for example, wouldn’t unduly shift your overall answer.
A new study led by Lukas Brunner at ETH Zurich uses an established method to weight the new model simulations based on how accurately they match the past few decades, as well as on how closely related each model is.
While the different climate models out there provide very beneficial independent checks, they’re not entirely independent. Some models were birthed from others, some share components, and some share methods. Dealing with this situation isn’t as simple as checking a GitHub fork history. In this case, the researchers analyze the spatial patterns of temperature and air pressure to calculate similarity between models. The more similar two models are, the more methods or code they are assumed to share, so each gets a little less influence in the overall average. This process minimizes the effect of double-counting models that aren’t really independent of each other.
Generally, the more important factor for weighting was the models’ ability to re-create the past. Obviously, models have to demonstrate skill in matching real-world data before you trust their projections of the future. All models are tested this way during their development, but they won’t end up with identical fits to the past—especially given that the past is complicated, so the models can be compared based on regional temperatures, precipitation, air pressure, and so on.
The researchers used five key properties from global datasets spanning 1980 to 2014. Each model was scored based on how accurately it matched temperatures, temperature trends, temperature variability, air pressures, and air pressure variability.
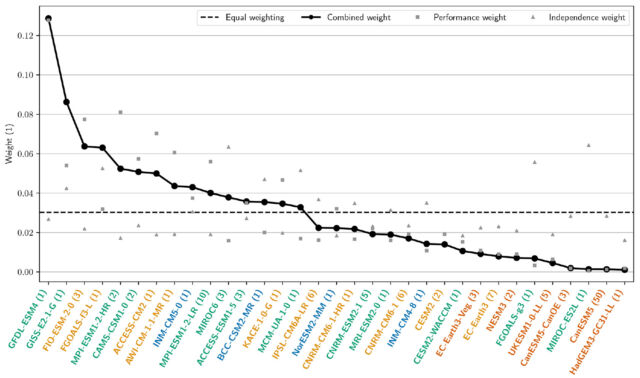
Enlarge / Here’s how the models were weighted—a simple average would have assigned each model equal weight (dashed line).
Don’t be so sensitive
This process ends up down-weighting the models with the highest climate sensitivity, as they don’t fit past observations as well. For example, using one metric of sensitivity called “Transient Climate Response,” the average before weighting is 2°C, with error bars spanning 1.6-2.5°C. But after weighting the models, the average drops slightly to 1.9°C, and the error bars shrink to 1.6-2.2°C. (That range lines up pretty nicely with recent estimates of the real value.)
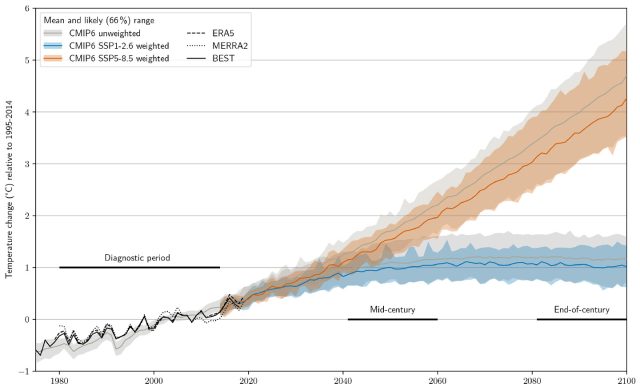
Enlarge / The gray line/shading shows the unweighted average projection of the models, but weighting drops the average and upper end a bit (orange for the high emissions scenario, blue for low).
Apply this to future projections of warming and something similar happens. In a high-emissions scenario, for example, warming between 2005 and 2090 goes from 4.1°C (3.1-4.9°C) down to 3.7°C (3.1-4.6°C). In the low-emissions scenario, average projected warming of 1.1°C (0.7-1.6°C) decreases to 1.0°C (0.7-1.4°C) after weighting.
The upshot here is that there’s no indication that the state of scientific knowledge has changed. As a consequence of ongoing development—particularly attempts to improve the realism of simulated clouds—some models became more sensitive to greenhouse gas increases, so they project stronger future warming. But it doesn’t seem to have made them better representations of Earth’s climate as a whole. Rather than worrying that the physics of climate change are even worse than we had thought, we can keep our focus on the urgent need to eliminate greenhouse gas emissions.
Earth System Dynamics, 2020. DOI: 10.5194/esd-11-995-2020 (About DOIs).