Researchers are using text mining and supervised machine learning to try and identify unique words and phrases in online posts that identify consumers’ interactions with hazardous food products.
The team of scientists from San Diego State University, Virginia Tech, Loyola Marymount University and Radford University, say they hope this will provide a practical and inexpensive means for rapidly monitoring food safety in real time.
These methods were used with a compiled data set of labeled consumer posts spanning two major websites. The researchers then compared their methods to traditional sentiment‐based text mining.
After assessing performance in a high‐volume setting, using a data set of more than 4 million online reviews, the study found its methods were 77 percent to 90 percent accurate in top‐ranking reviews, while sentiment analysis was just 11 percent to 26 percent accurate. The study also aggregated review‐level results to make product‐level risk assessments.
A panel of 21 food safety experts assessed the model’s ability to identify products that exhibit a substantially higher risk than baseline products.
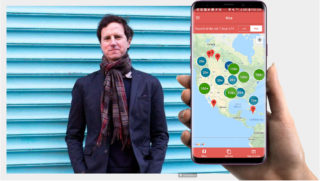
The researchers said Patrick Quade, founder of iwaspoisoned.com, provided access to a data set of food safety reports that made the study possible. The iwaspoisoned.com platform is a consumer led website for diners to report suspected food poisoning or bad food experiences.
Quade’s website has public health subscribers in 45 U.S. states. On the world level, public health departments from Singapore, the United Kingdom, Canada, Australia and Germany subscribe to the iwaspoisoned.com alert service.
The website has been credited with helping to identify several high-profile foodborne illness outbreaks in recent years.
More information on this study can be found here.
(To sign up for a free subscription to Food Safety News, click here)